Back
Is data really all you need?
Boost Model Accuracy by Mastering Data Preparation and Curation
Aug 1, 2024
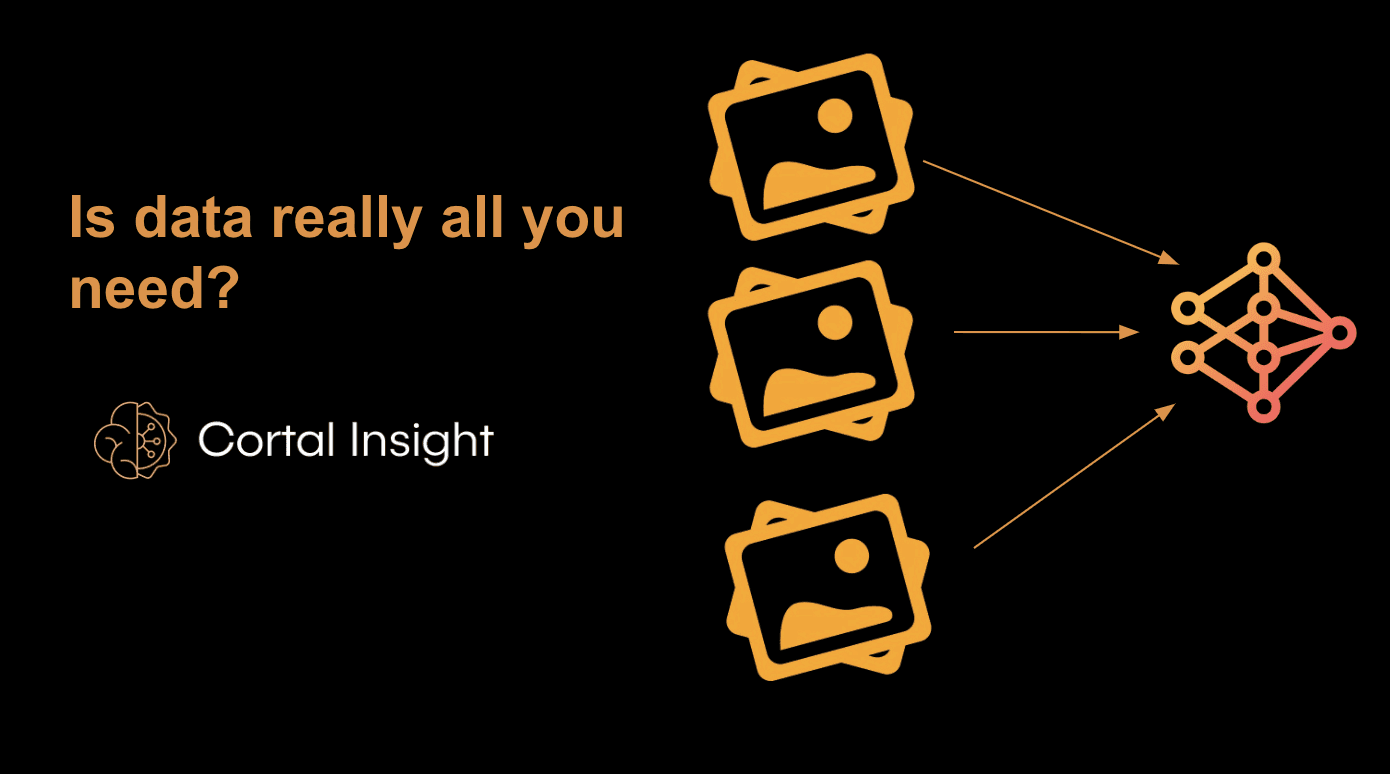
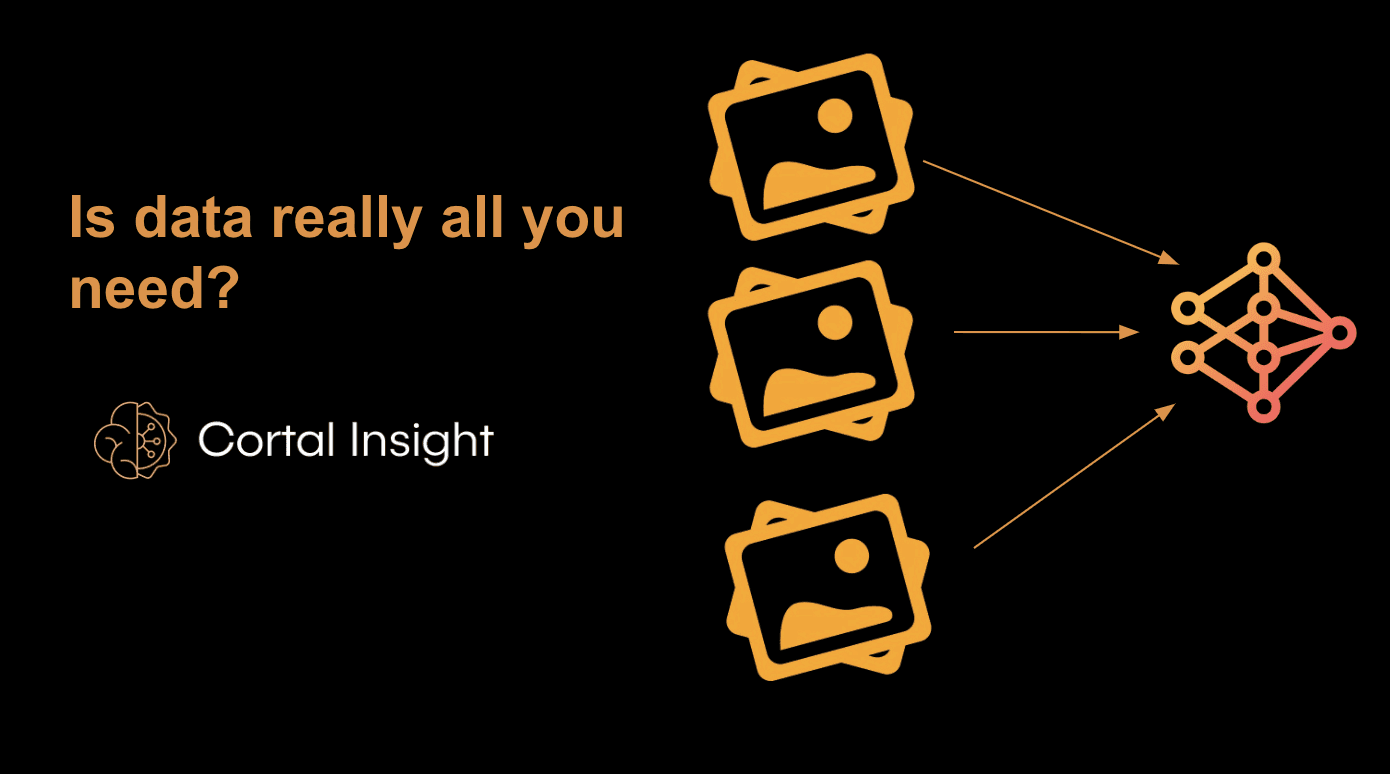
Recent breakthroughs in AI, particularly in Computer Vision, have led to significant advancements and widespread adoption in the field. Computer vision is extremely powerful when done right. One of the most cliched statements has never been more relevant ‘Garbage in -> Garbage out’.
Most AI experiments follow four key phases:
Defining the problem,
Preparing the data,
Training the model, and
Building the application.
Data preparation often consumes the most time. This process involves several critical steps: exploratory data analysis, data curation, data annotation, and data preprocessing. Despite its importance, data preparation can be incredibly hard and time-consuming. You are only as successful as mastery of your data, simply adding more data or using a more complex model won't necessarily improve your results.
At Cortal Insight, we aim to enable machine learning teams to focus more on innovation and less on routine data practices.
Here’s how we can help:
Eliminate low-quality data: Filter out any data that could negatively impact your models. This includes images that are blurry, too dark, too light, or noisy
Assess Data Health: Regularly evaluate the overall health and composition of your dataset. Understanding your data's current state helps in identifying areas for improvement
Configure Data Audits: Customize data audits based on your specific requirements to ensure only the most relevant data is used.
Identify and Remove Outliers: Improve the reliability of your datasets by detecting and removing outliers and redundant samples.
Enhance Data Diversity: Analyze patterns and relationships within your data to ensure it is diverse and well-suited for its intended purpose.
Curate Your Dataset Efficiently: Simplify the process of data curation with a single line command.
Connect with our team at Cortal Insight, who've cracked some of the world's most complex problems. Schedule a consultation to discover how our platform can help your machine learning teams to accelerate your experiments and deploy your models faster.
Recent breakthroughs in AI, particularly in Computer Vision, have led to significant advancements and widespread adoption in the field. Computer vision is extremely powerful when done right. One of the most cliched statements has never been more relevant ‘Garbage in -> Garbage out’.
Most AI experiments follow four key phases:
Defining the problem,
Preparing the data,
Training the model, and
Building the application.
Data preparation often consumes the most time. This process involves several critical steps: exploratory data analysis, data curation, data annotation, and data preprocessing. Despite its importance, data preparation can be incredibly hard and time-consuming. You are only as successful as mastery of your data, simply adding more data or using a more complex model won't necessarily improve your results.
At Cortal Insight, we aim to enable machine learning teams to focus more on innovation and less on routine data practices.
Here’s how we can help:
Eliminate low-quality data: Filter out any data that could negatively impact your models. This includes images that are blurry, too dark, too light, or noisy
Assess Data Health: Regularly evaluate the overall health and composition of your dataset. Understanding your data's current state helps in identifying areas for improvement
Configure Data Audits: Customize data audits based on your specific requirements to ensure only the most relevant data is used.
Identify and Remove Outliers: Improve the reliability of your datasets by detecting and removing outliers and redundant samples.
Enhance Data Diversity: Analyze patterns and relationships within your data to ensure it is diverse and well-suited for its intended purpose.
Curate Your Dataset Efficiently: Simplify the process of data curation with a single line command.
Connect with our team at Cortal Insight, who've cracked some of the world's most complex problems. Schedule a consultation to discover how our platform can help your machine learning teams to accelerate your experiments and deploy your models faster.
Recent breakthroughs in AI, particularly in Computer Vision, have led to significant advancements and widespread adoption in the field. Computer vision is extremely powerful when done right. One of the most cliched statements has never been more relevant ‘Garbage in -> Garbage out’.
Most AI experiments follow four key phases:
Defining the problem,
Preparing the data,
Training the model, and
Building the application.
Data preparation often consumes the most time. This process involves several critical steps: exploratory data analysis, data curation, data annotation, and data preprocessing. Despite its importance, data preparation can be incredibly hard and time-consuming. You are only as successful as mastery of your data, simply adding more data or using a more complex model won't necessarily improve your results.
At Cortal Insight, we aim to enable machine learning teams to focus more on innovation and less on routine data practices.
Here’s how we can help:
Eliminate low-quality data: Filter out any data that could negatively impact your models. This includes images that are blurry, too dark, too light, or noisy
Assess Data Health: Regularly evaluate the overall health and composition of your dataset. Understanding your data's current state helps in identifying areas for improvement
Configure Data Audits: Customize data audits based on your specific requirements to ensure only the most relevant data is used.
Identify and Remove Outliers: Improve the reliability of your datasets by detecting and removing outliers and redundant samples.
Enhance Data Diversity: Analyze patterns and relationships within your data to ensure it is diverse and well-suited for its intended purpose.
Curate Your Dataset Efficiently: Simplify the process of data curation with a single line command.
Connect with our team at Cortal Insight, who've cracked some of the world's most complex problems. Schedule a consultation to discover how our platform can help your machine learning teams to accelerate your experiments and deploy your models faster.
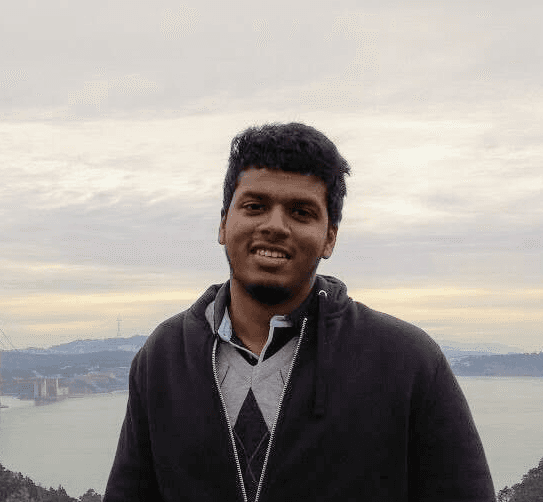
Preetham Rajkumar
Other articles you might like
Learn how to increase your productivity with calendars