Back
Save Money on Annotation - Pick Only Images That Matter
Cut Annotation Costs with Smarter Data Selection
Sep 4, 2024
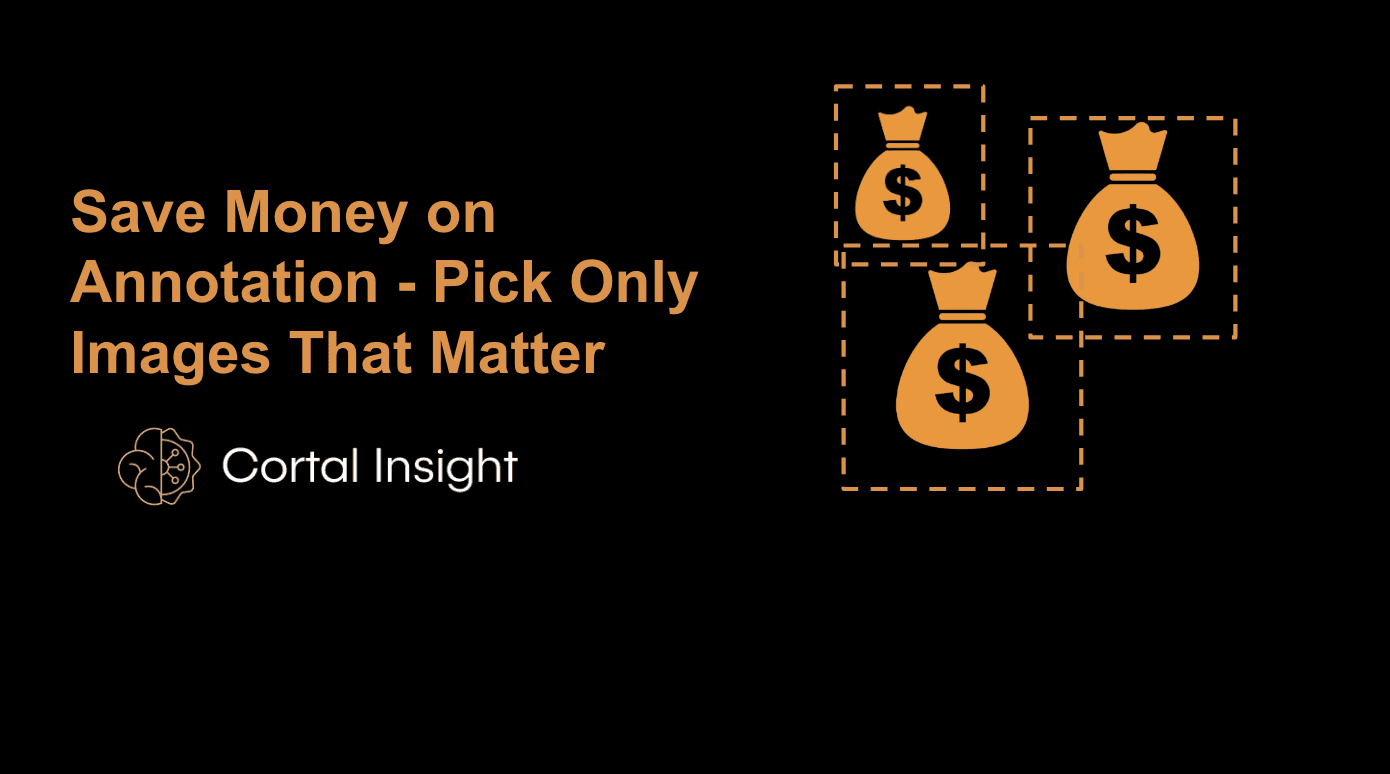
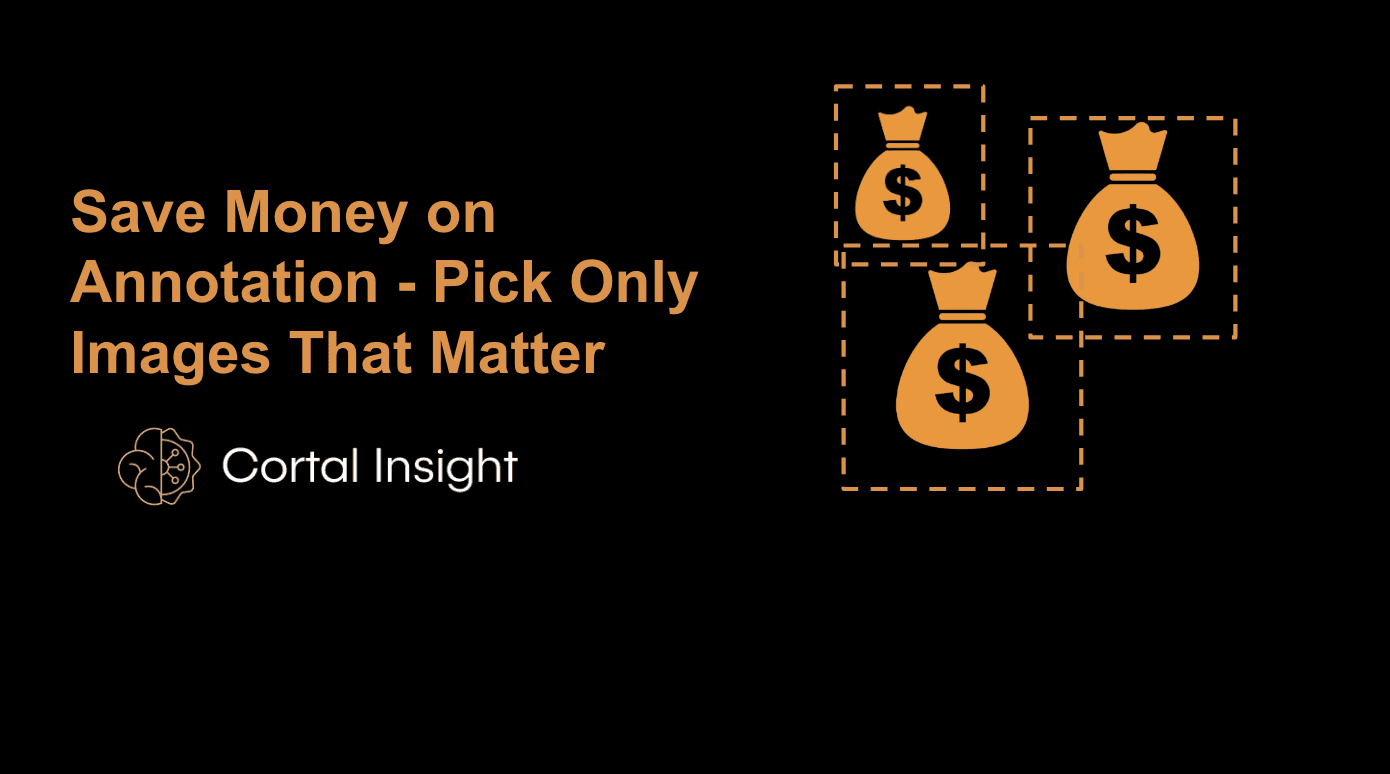
In the fast-paced world of machine learning, particularly for SMBs, every dollar counts. Apart from cloud training, area where costs can quickly spiral out of control is data annotation
Their process often involves annotating vast amounts of data—specifically images—to train models. However, not all images are created equal, and annotating every image can quickly become a costly endeavor.
At Cortal Insight, we believe that effective data management can significantly cut down annotation costs by ensuring that only the most valuable images are chosen for annotation.
Hidden Costs of Annotating Every Image
Annotation is utmost important for training computer vision models. But imagine this: you've collected thousands of images from your manufacturing floor or retail store cameras and this needs to be annotated to train your model. Many ML teams fall into the trap of annotating every image in their dataset.
If you're not selective, you'll end up spending valuable resources—both time and money—on annotating images that are blurry, poorly lit, or irrelevant.
Prioritize High-Quality, Relevant Images
Instead of dumping all your raw data into the annotation pipeline, you can save money and time by selecting only those images that truly matter. Here's how Cortal Insight can help:
1. Data Quality Checks
Before you spend a single cent on annotation, it’s crucial to weed out low-quality images that do not contribute to model accuracy. Cortal Insight automatically identifies and filters out images that are:
Blurry: Out-of-focus images can lead to poor model training and misclassification.
Dark or Bright: Images with extreme lighting conditions might obscure critical features that are important for model training.
Noisy: Excessive noise in images can distort true data signals, confusing your model during the training process.
By eliminating these low-quality images early on, you focus your annotation efforts on high-quality data, directly reducing costs and enhancing model performance.
2. Data Audit Checks
Redundant data can bloat your dataset and unnecessarily increase annotation costs. Cortal Insight performs comprehensive data audits to remove:
Duplicates and Near-Duplicates: Annotating the same or near-similar images multiple times adds no value but significantly increases costs.
Outliers: Images that deviate significantly from the norm can mislead model training if not properly managed.
Removing redundant and irrelevant data not only saves on annotation costs but also prevents your model from overfitting on redundant data.
3. Data Analytics for Balanced Datasets
Another critical aspect of saving on annotation costs is ensuring your dataset is balanced and diverse. For instance, if your ML model is trained to detect product defects in a manufacturing line, an unbalanced dataset could lead to biased model performance. We provide insights on:
Assess Class Balance: Identify if your dataset is skewed towards certain classes and adjust accordingly to ensure even representation.
Evaluate Image Resolution: Ensure all images meet a standard resolution, so no annotation effort is wasted on subpar images.
With these analytics, you can make informed decisions about which images to prioritize for annotation.
The Benefits: Beyond Cost Savings
Immediate benefit is – reduced annotation costs – the advantages extend further:
Faster Time-to-Market: By focusing on only the most relevant images, you can complete your annotation process more quickly.
Improved Model Performance: A cleaner, more balanced dataset leads to better-performing models.
Resource Optimization: Your team can focus on high-value tasks instead of managing redundant data.
Conclusion: Let Your Data Work Smarter, Not Harder
For ML teams, especially those operating within the constraints of SMBs or startups, every dollar counts. Don't let unnecessary annotation costs hold your startup back. Start picking only the images that matter, and watch your ML projects accelerate while your costs decrease.
At Cortal Insight, our data management platform helps you achieve this level of efficiency, automating day-to-day data operations and enabling your ML team to accelerate their experiments through better data preparedness.
In the fast-paced world of machine learning, particularly for SMBs, every dollar counts. Apart from cloud training, area where costs can quickly spiral out of control is data annotation
Their process often involves annotating vast amounts of data—specifically images—to train models. However, not all images are created equal, and annotating every image can quickly become a costly endeavor.
At Cortal Insight, we believe that effective data management can significantly cut down annotation costs by ensuring that only the most valuable images are chosen for annotation.
Hidden Costs of Annotating Every Image
Annotation is utmost important for training computer vision models. But imagine this: you've collected thousands of images from your manufacturing floor or retail store cameras and this needs to be annotated to train your model. Many ML teams fall into the trap of annotating every image in their dataset.
If you're not selective, you'll end up spending valuable resources—both time and money—on annotating images that are blurry, poorly lit, or irrelevant.
Prioritize High-Quality, Relevant Images
Instead of dumping all your raw data into the annotation pipeline, you can save money and time by selecting only those images that truly matter. Here's how Cortal Insight can help:
1. Data Quality Checks
Before you spend a single cent on annotation, it’s crucial to weed out low-quality images that do not contribute to model accuracy. Cortal Insight automatically identifies and filters out images that are:
Blurry: Out-of-focus images can lead to poor model training and misclassification.
Dark or Bright: Images with extreme lighting conditions might obscure critical features that are important for model training.
Noisy: Excessive noise in images can distort true data signals, confusing your model during the training process.
By eliminating these low-quality images early on, you focus your annotation efforts on high-quality data, directly reducing costs and enhancing model performance.
2. Data Audit Checks
Redundant data can bloat your dataset and unnecessarily increase annotation costs. Cortal Insight performs comprehensive data audits to remove:
Duplicates and Near-Duplicates: Annotating the same or near-similar images multiple times adds no value but significantly increases costs.
Outliers: Images that deviate significantly from the norm can mislead model training if not properly managed.
Removing redundant and irrelevant data not only saves on annotation costs but also prevents your model from overfitting on redundant data.
3. Data Analytics for Balanced Datasets
Another critical aspect of saving on annotation costs is ensuring your dataset is balanced and diverse. For instance, if your ML model is trained to detect product defects in a manufacturing line, an unbalanced dataset could lead to biased model performance. We provide insights on:
Assess Class Balance: Identify if your dataset is skewed towards certain classes and adjust accordingly to ensure even representation.
Evaluate Image Resolution: Ensure all images meet a standard resolution, so no annotation effort is wasted on subpar images.
With these analytics, you can make informed decisions about which images to prioritize for annotation.
The Benefits: Beyond Cost Savings
Immediate benefit is – reduced annotation costs – the advantages extend further:
Faster Time-to-Market: By focusing on only the most relevant images, you can complete your annotation process more quickly.
Improved Model Performance: A cleaner, more balanced dataset leads to better-performing models.
Resource Optimization: Your team can focus on high-value tasks instead of managing redundant data.
Conclusion: Let Your Data Work Smarter, Not Harder
For ML teams, especially those operating within the constraints of SMBs or startups, every dollar counts. Don't let unnecessary annotation costs hold your startup back. Start picking only the images that matter, and watch your ML projects accelerate while your costs decrease.
At Cortal Insight, our data management platform helps you achieve this level of efficiency, automating day-to-day data operations and enabling your ML team to accelerate their experiments through better data preparedness.
In the fast-paced world of machine learning, particularly for SMBs, every dollar counts. Apart from cloud training, area where costs can quickly spiral out of control is data annotation
Their process often involves annotating vast amounts of data—specifically images—to train models. However, not all images are created equal, and annotating every image can quickly become a costly endeavor.
At Cortal Insight, we believe that effective data management can significantly cut down annotation costs by ensuring that only the most valuable images are chosen for annotation.
Hidden Costs of Annotating Every Image
Annotation is utmost important for training computer vision models. But imagine this: you've collected thousands of images from your manufacturing floor or retail store cameras and this needs to be annotated to train your model. Many ML teams fall into the trap of annotating every image in their dataset.
If you're not selective, you'll end up spending valuable resources—both time and money—on annotating images that are blurry, poorly lit, or irrelevant.
Prioritize High-Quality, Relevant Images
Instead of dumping all your raw data into the annotation pipeline, you can save money and time by selecting only those images that truly matter. Here's how Cortal Insight can help:
1. Data Quality Checks
Before you spend a single cent on annotation, it’s crucial to weed out low-quality images that do not contribute to model accuracy. Cortal Insight automatically identifies and filters out images that are:
Blurry: Out-of-focus images can lead to poor model training and misclassification.
Dark or Bright: Images with extreme lighting conditions might obscure critical features that are important for model training.
Noisy: Excessive noise in images can distort true data signals, confusing your model during the training process.
By eliminating these low-quality images early on, you focus your annotation efforts on high-quality data, directly reducing costs and enhancing model performance.
2. Data Audit Checks
Redundant data can bloat your dataset and unnecessarily increase annotation costs. Cortal Insight performs comprehensive data audits to remove:
Duplicates and Near-Duplicates: Annotating the same or near-similar images multiple times adds no value but significantly increases costs.
Outliers: Images that deviate significantly from the norm can mislead model training if not properly managed.
Removing redundant and irrelevant data not only saves on annotation costs but also prevents your model from overfitting on redundant data.
3. Data Analytics for Balanced Datasets
Another critical aspect of saving on annotation costs is ensuring your dataset is balanced and diverse. For instance, if your ML model is trained to detect product defects in a manufacturing line, an unbalanced dataset could lead to biased model performance. We provide insights on:
Assess Class Balance: Identify if your dataset is skewed towards certain classes and adjust accordingly to ensure even representation.
Evaluate Image Resolution: Ensure all images meet a standard resolution, so no annotation effort is wasted on subpar images.
With these analytics, you can make informed decisions about which images to prioritize for annotation.
The Benefits: Beyond Cost Savings
Immediate benefit is – reduced annotation costs – the advantages extend further:
Faster Time-to-Market: By focusing on only the most relevant images, you can complete your annotation process more quickly.
Improved Model Performance: A cleaner, more balanced dataset leads to better-performing models.
Resource Optimization: Your team can focus on high-value tasks instead of managing redundant data.
Conclusion: Let Your Data Work Smarter, Not Harder
For ML teams, especially those operating within the constraints of SMBs or startups, every dollar counts. Don't let unnecessary annotation costs hold your startup back. Start picking only the images that matter, and watch your ML projects accelerate while your costs decrease.
At Cortal Insight, our data management platform helps you achieve this level of efficiency, automating day-to-day data operations and enabling your ML team to accelerate their experiments through better data preparedness.
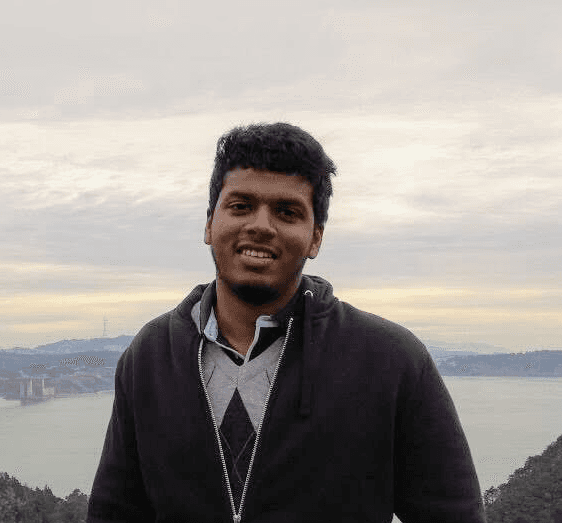
Preetham Rajkumar
Other articles you might like
Learn how to increase your productivity with calendars